Iu Ayala from Gradient Insight shares his insights on how AI-driven computer vision is revolutionizing industries like manufacturing, retail, and logistics. In manufacturing, AI is enhancing quality control by detecting defects in real time and improving worker safety through hazard recognition. Retailers are leveraging AI-powered cameras for automated inventory tracking and checkout-free shopping experiences, while also analyzing customer behavior to optimize store layouts. Logistics and supply chains benefit from AI vision through real-time shipment tracking, automated sorting, and enhanced warehouse efficiency. As AI continues to evolve, businesses that integrate these technologies will gain a competitive edge by reducing costs, improving accuracy, and driving automation at scale.
How will AI-driven computer vision change industries like manufacturing, retail, and logistics?
AI-driven computer vision is already reshaping industries by bringing intelligence and automation to processes that used to rely heavily on manual effort. In manufacturing, companies are using AI to detect product defects in real time, ensuring that faulty items don’t make it through the production line. This not only improves quality control but also reduces waste and saves money. Some factories are even integrating AI-powered cameras that monitor worker safety, preventing accidents by recognizing hazards before they become problems.
Retail is also undergoing a major shift. Instead of relying on employees to track inventory manually, AI-powered cameras can scan shelves and alert staff when stock is running low. Some retailers are experimenting with fully automated checkout systems that recognize products as customers walk out, eliminating the need for traditional checkouts. Beyond inventory management, AI vision is also being used to analyze customer behavior in stores, helping businesses optimize layouts and improve sales strategies.
Logistics and supply chain operations are seeing some of the most significant transformations. AI-powered tracking systems can now visually monitor shipments as they move through warehouses and across the globe, reducing losses and improving efficiency. In large distribution centers, robots with computer vision are automating tasks like sorting and packaging, speeding up operations while reducing errors. As AI vision continues to improve, businesses that invest in these technologies will gain a huge competitive advantage, cutting costs and improving accuracy across their entire workflow.
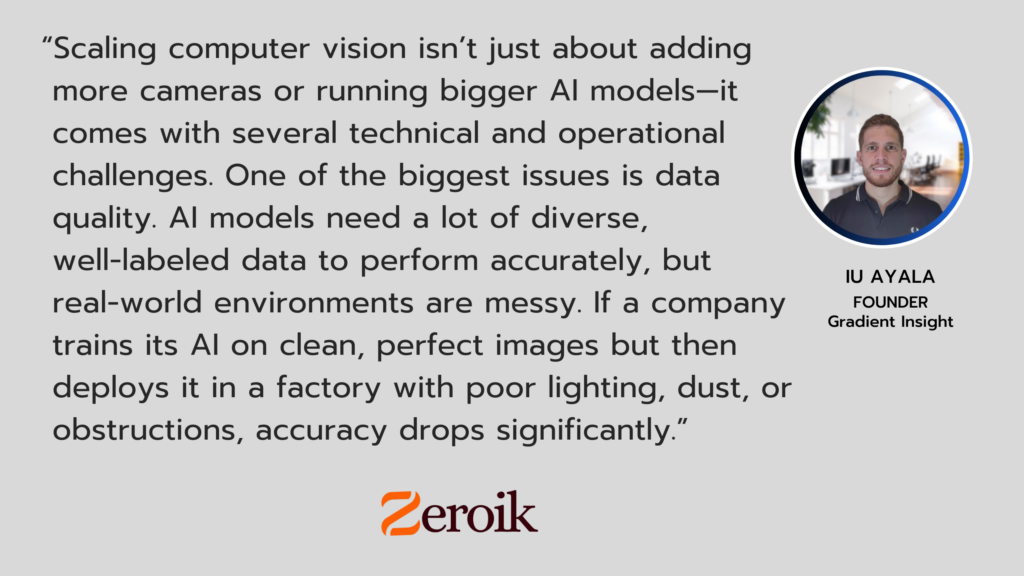
How can combining vision, language, and audio improve real-world AI applications?
AI systems are becoming much more powerful by combining different types of data—vision, language, and audio—into a single, cohesive model. When AI can “see,” “hear,” and “understand” at the same time, it becomes far more useful in real-world scenarios.
For example, imagine a customer support system where an AI assistant can analyze a live video feed of a broken product, recognize the issue, and simultaneously process what the customer is saying. If the AI also listens to the customer’s tone of voice, it can gauge frustration levels and adjust its response accordingly, making interactions feel much more human and helpful. This kind of technology is particularly useful for remote troubleshooting, where AI can visually diagnose issues and provide guided solutions in real time.
In industrial settings, multimodal AI can improve automation and worker collaboration. A warehouse worker could verbally instruct an AI system to locate and retrieve a package, while the AI uses its vision capabilities to track down the exact item. AI-powered robots that combine vision and audio can also work more effectively alongside human employees, responding to both visual cues and spoken commands.
The future of AI isn’t just about making machines better at one task—it’s about creating systems that can process and integrate information the way humans do. Businesses that leverage this kind of technology will unlock entirely new ways to improve efficiency, customer experience, and automation.
What are the biggest challenges in using computer vision at scale?
Scaling computer vision isn’t just about adding more cameras or running bigger AI models—it comes with several technical and operational challenges. One of the biggest issues is data quality. AI models need a lot of diverse, well-labeled data to perform accurately, but real-world environments are messy. If a company trains its AI on clean, perfect images but then deploys it in a factory with poor lighting, dust, or obstructions, accuracy drops significantly.
Another major challenge is real-time processing. Many industries require AI to analyze visual data instantly, but that requires serious computing power. Edge computing—where AI processes data locally instead of sending everything to the cloud—can help, but it’s not always easy to implement. Businesses need to balance accuracy with speed while keeping infrastructure costs under control.
Security and privacy are also critical concerns, especially in customer-facing industries like retail. AI vision systems that track foot traffic or monitor employees need to handle data responsibly, complying with regulations and ensuring transparency. Companies that want to scale AI vision successfully need to have a strategy for data governance, model accuracy, and computational efficiency.
How do you maintain high accuracy when dealing with low-quality or messy visual data?
Dealing with low-quality or inconsistent visual data is one of the biggest challenges in computer vision, but there are ways to overcome it. One of the most effective techniques is data preprocessing. AI models can be trained to clean up images before analysis by using noise reduction, contrast enhancement, or even AI-based super-resolution techniques that improve clarity. This helps the AI extract useful information even from low-quality images.
Another important strategy is using domain-specific AI models instead of generic ones. A general image recognition model might struggle with blurry security camera footage in a warehouse, but a model trained specifically on that environment will perform much better. Fine-tuning AI with data from real-world conditions is crucial to maintaining accuracy.
Sometimes, the best solutions are physical rather than algorithmic. Improving camera placement, upgrading lighting, or switching to higher-resolution sensors can significantly boost AI performance. Businesses that combine technical fixes with smart hardware choices will get the most out of their computer vision systems, even in challenging environments.
What are the main difficulties in combining computer vision with robotics and automation?
Bringing computer vision into robotics and automation sounds straightforward, but it’s full of challenges. One of the biggest problems is dealing with real-world variability. Robots work great in structured environments where everything is predictable, but the moment something changes—like an object being slightly out of place or lighting conditions shifting—the AI has to adapt. Training robots to handle unpredictable conditions requires a lot of high-quality data and advanced machine learning techniques.
Latency is another major issue. Robots that rely on computer vision need to make split-second decisions, whether it’s avoiding an obstacle or grabbing an object with precision. Processing visual data in real-time requires powerful hardware and efficient algorithms, and any delay can reduce performance or even cause failures.
Integration with existing systems is another hurdle. Many warehouses and factories still use older machines that weren’t designed with AI in mind. Retrofitting these systems to work with AI-powered vision requires careful planning and investment. Businesses that want to integrate AI into their robotics workflows should start with phased implementations, testing systems in controlled environments before rolling them out on a larger scale.
What major improvements do you expect in AI-powered inspection for warehouses and supply chains?
AI-powered inspection systems are becoming much more sophisticated, and we’re going to see some major improvements in the near future. One of the biggest advancements will be fully automated quality control. Instead of just flagging defects, AI will start predicting them before they happen. By analyzing patterns in production data, AI can anticipate when a machine is likely to produce faulty products and recommend maintenance before problems arise.
Another exciting development is the use of 3D vision systems. Traditional AI vision systems struggle with depth perception, but new 3D camera technologies are allowing AI to see objects in much greater detail. This means more accurate inspections, whether it’s checking for tiny defects in manufactured parts or verifying the integrity of packages in a warehouse.
AI-powered tracking will also become far more precise, reducing errors in supply chain management. Cameras and sensors will be able to track every item in real-time, ensuring that nothing gets lost or misplaced. This will lead to better inventory control, faster order fulfillment, and fewer operational bottlenecks. Businesses that invest in these AI-driven inspection tools will see big improvements in efficiency, cost savings, and overall supply chain reliability.