In this interview, Yuliia Koinak, CEO and Founder of LAB325, discusses key AI challenges and how her company is solving them. Drawing on her IT and healthcare expertise, Yuliia highlights strategies for overcoming data issues, resistance to change, and unrealistic expectations, while promoting AI solutions like multi-agent systems and edge computing. She also addresses common AI myths and emphasizes the importance of fairness, transparency, and accountability. Yuliia’s work in AI-driven prosthetics rehabilitation and personalized healthcare showcases her commitment to improving health outcomes globally. This interview offers practical insights into AI’s transformative potential in various industries.
What are the most important factors for ensuring a smooth AI adoption process at the enterprise level?
In our experience, the most important factor for successful AI adoption is a clear understanding of the risks associated with integrating AI into business processes. AI solutions can significantly assist employees, automate routine tasks, and even take over certain responsibilities. However, it’s crucial to remember that AI systems, no matter how advanced, are not immune to errors.
The more complex the AI system, the harder it becomes to predict and prevent potential mistakes. Therefore, enterprises must:
- Carefully monitor decisions made by AI, especially in critical processes.
- Always assume that the system could fail or produce an incorrect outcome at some point.
- Implement human oversight mechanisms at key decision points to minimize risks. Additionally, we often find that business leaders underestimate the true cost of implementing and using AI. While subscription-based services like ChatGPT might appear inexpensive, active API usage can lead to substantial expenses. Building custom AI models is even more resource-intensive, often requiring hundreds of thousands of dollars for infrastructure, data preparation, specialist expertise, and raining. Enterprises need to evaluate not only the benefits but also the long-term costs of maintaining and scaling these solutions to avoid unexpected financial burdens.
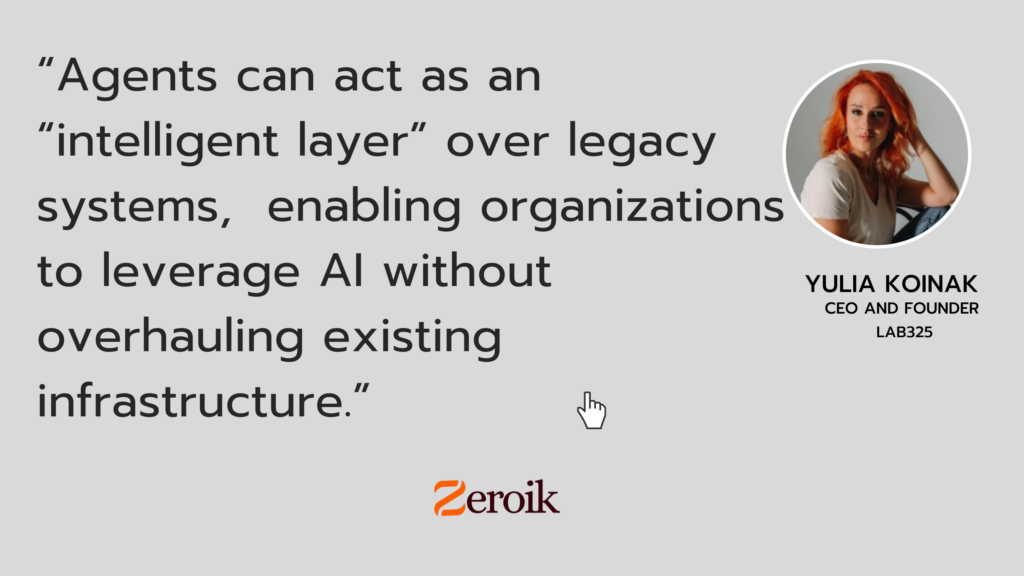
What are the common challenges your clients face when implementing AI, and how do you overcome them?
- Lack of data: Many clients struggle with insufficient or poorly structured data. We assist in developing strategies for data collection, cleaning, and augmentation to address these gaps.
- Resistance to change: Organizations sometimes face resistance from employees or leadership. We conduct educational sessions to demonstrate the benefits of AI and how it enhances—not replaces—human work.
- Unrealistic expectations: Clients often view AI as a “magic bullet.” We set realistic expectations by explaining that achieving meaningful results requires time, robust data, and iterative testing.
- Ethical and regulatory concerns: Industries like healthcare and finance face strict compliance requirements. We help clients build transparent and explainable models to address these concerns.
- Lack of expertise: Many organizations lack internal AI expertise. We provide end-to-end development, from model creation to integration, to bridge this gap.
Are there any emerging AI trends or technologies that you’re particularly excited about? How will they shape industries like manufacturing, healthcare, or finance?
- Self-improving systems: Multi-agent systems often include mechanisms for continuous learning, allowing agents to adapt and improve over time. This is crucial for real-time applications in manufacturing and healthcare.
- AI + Edge Computing: Combining AI with edge computing allows for low-latency, privacy-preserving solutions, particularly valuable in industries like finance and manufacturing.
- Integration with traditional software: Agents can act as an “intelligent layer” over legacy systems, enabling organizations to leverage AI without overhauling existing infrastructure.
Impact on industries:
- Healthcare: Multi-agent systems can coordinate diagnostics, treatment planning, and patient monitoring. For instance, one agent might analyze medical images, while another monitors patient data, with a manager-agent synthesizing the insights.
- Manufacturing: These systems automate complex production chains, optimize resource usage, and enhance quality control.
- Finance: Multi-agent systems excel in risk management, portfolio optimization, and real-time market analysis, ensuring speed and precision.
What are some common misconceptions about AI that you encounter when working with new clients, and how do you address them?
- “AI will replace all employees“: We explain that AI is a tool designed to automate repetitive tasks, not replace people. It enables employees to focus on more complex and impactful activities.
- “AI solves all problems instantly“: We emphasize that successful AI projects require time, data preparation, and iterative model improvement.
- “AI is simple“: A common misconception is that implementing AI is straightforward and inexpensive. On the contrary, it is often a complex and costly process involving high-quality data, advanced infrastructure, skilled professionals, and ongoing maintenance. We candidly discuss the financial and technical realities to help clients make informed decisions.
- “AI is always accurate“: We highlight that AI models are only as good as the data they are trained on. Poor data quality or unclear problem definitions can lead to errors, underscoring the need for careful design and validation.
By addressing these misconceptions, we help clients set realistic expectations and develop
practical, impactful AI solutions.
How do you ensure AI models are fair, transparent, and accountable, especially when dealing with sensitive industries such as healthcare or finance?
- Ethical decision-making: Our solutions are designed to align with ethical standards, ensuring that AI serves as a tool to support, not replace, human expertise in critical fields like healthcare. These practices enable us to build trustworthy, reliable AI systems that drive meaningful impact while safeguarding fairness and accountability.
- Explainability: We use Explainable AI (XAI) techniques to ensure that models’ decisions are interpretable and transparent for stakeholders, regulators, and end-users.
- Fairness: We analyze training data to identify and mitigate biases, ensuring that models
make fair and equitable decisions. - Working with sensitive data: In industries like healthcare and finance, sensitive data often cannot be used directly due to privacy concerns. To address this, we generate synthetic data that retains the statistical and structural properties of real data without exposing any personally identifiable information. This allows us to train models effectively while fully complying with regulations like GDPR and HIPAA.
- Regular testing and audits: We rigorously test models on diverse datasets, including edge cases, to ensure consistent performance across scenarios.
- Accountability: We implement logging and monitoring systems to track model decisions and inputs, enabling retrospective analysis and error detection.